New approach improves the prediction accuracy of agronomically relevant traits
Gatersleben/ Potsdam - Advances in high-throughput phenotyping (HTP) platforms together with genotyping technologies have revolutionized breeding of varieties with desired traits relying on genomic prediction. Yet, we lack understanding of the expression of multiple traits at different time points across the entire growth period of the plant. A research team, including IPK Leibniz Institue, the Max Planck Institute of Molecular Plant Physiology and the University of Potsdam, has developed a computational approach to solve this problem. The results were published in the journal “Nature Plants”.
The phenome of a plant comprises the entirety of traits expressed at any given time, and is the integrated outcome of the effects of genetic factors, environmental conditions and their complex interactions. Understanding how the crop phenome changes over time can help predict individual traits at specific time points in crop development. However, this problem is challenging not only because of the intricate dependence between individual traits, but also due to differences in how the phenomes of specific genotypes change over the plant life cycle.
The classical approach of genomic prediction (GP) in crops trains machine learning models using data on traits measured in a population of genotypes at a specific time point based on genetic markers. However, existing GP approaches have not yet addressed the problem of predicting the dynamics of multiple traits, i.e., the expression of multiple traits at different time points across the entire growth period of the plant.
The research team introduced dynamicGP, a computational approach that facilitates the prediction of trait dynamics across development in crops for which time-series phenotypic measurements for multiple genotypes are available from HTP platforms. “We demonstrated that dynamicGP is an efficient computational approach to predict genotype-specific dynamics for multiple traits. This is achieved by combining genomic prediction with dynamic mode decomposition (DMD)”, says David Hobby, researcher at the Max Planck Institute of Molecular Plant Physiology and the University of Potsdam and one of the first authors of the study.
Using genetic markers and data from high-throughput phenotyping of a maize multi-parent advanced generation inter-cross population and an Arabidopsis thaliana diversity panel, the researchers showed that dynamicGP outperforms a state-of-the-art genomic prediction approach for multiple traits. “We found that the developmental dynamics of traits whose heritability varies less over time can be predicted with higher accuracy, shedding light on a factor that affect the predictability of traits over developmental trajectory”, says Dr. Marc Heuermann, researcher at the IPK Leibniz Institute and also one of the first authors of the study.
Therefore, dynamicGP paves the way for interrogating and integrating the dynamical interactions between genotype and phenotype over crop development to improve the prediction accuracy of agronomically relevant traits. Future developments of dynamicGP can rely on extensions of DMD to consider effects of environmental factors. These will facilitate further refinements of the proposed approach that are expected to have very substantial impact on breeding crop varieties adapted to particular regions as well as precision agriculture.
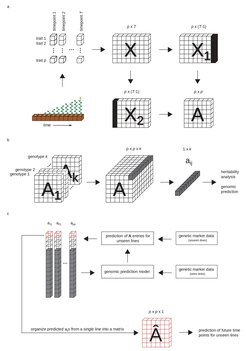
a) Data on phenotypic traits (p) of a genotype are collected over time (T) and utilized to find a time-invariant best-fit linear operator (A) that transforms the phenome from one time point to the next. b) When time-resolved data are available for multiple (k) genotypes, we obtain an p x p x k tensor whose elements can be treated as traits in heritability analyses and genomic prediction. c) Then, models for unseen lines can be trained using their genetic information and applied to predict future time points for the line.
Joint press release of the IPK Leibniz Institute and the Max Planck Institute of Molecular Plant Physiology.